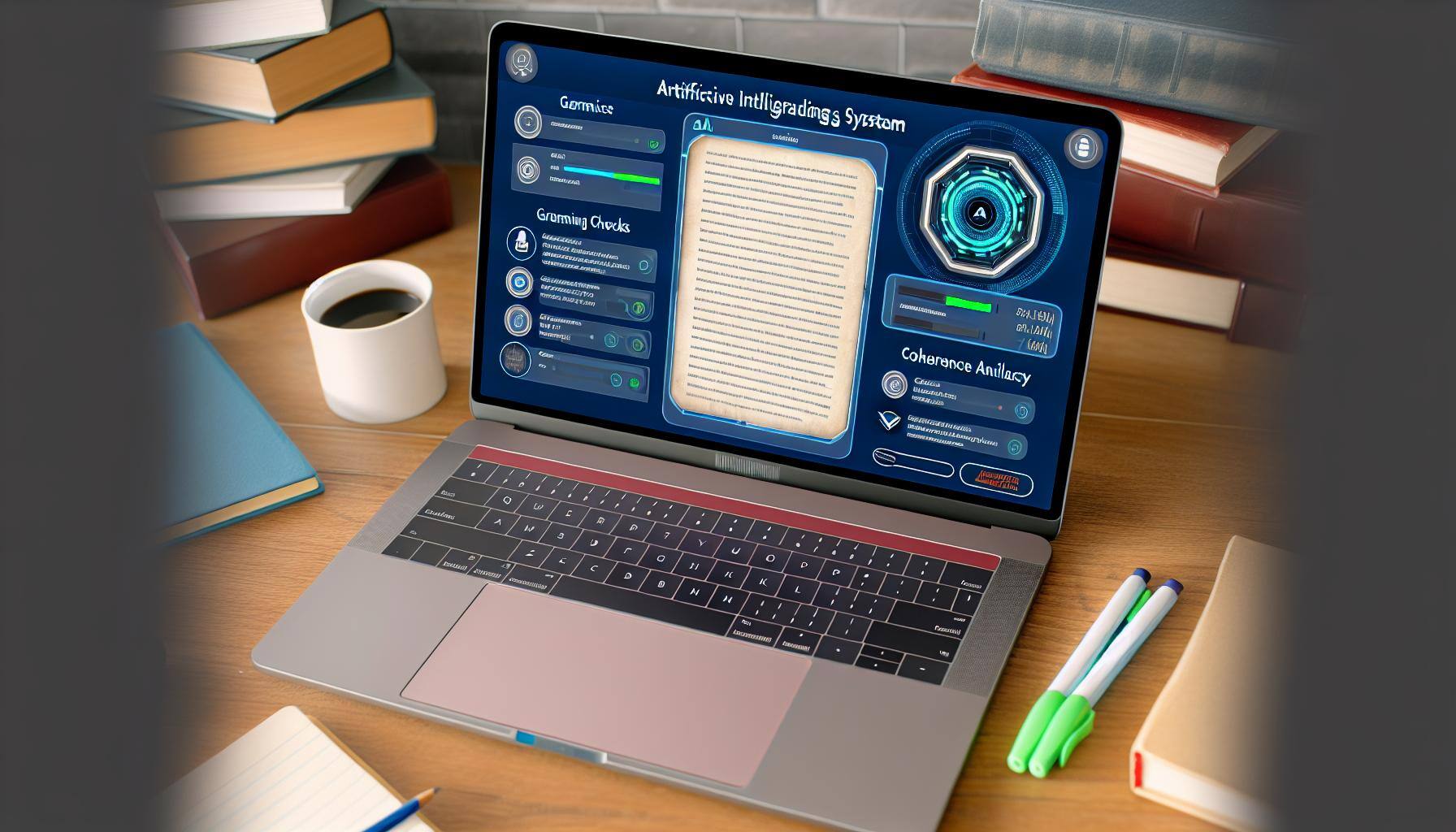
Artificial Intelligence (AI) has made significant inroads into various educational processes, including the grading of assignments. It can be an effective way to grade multiple choice, and even some short answer, questions - but when it comes to long-form writing assignments, things can get a bit tricky.
Read on to learn about the limitations facing AI when it comes to assessing long-form writing.
1. Interpretation and Contextual Understanding
One of the primary limitations of AI in grading long-form writing assignments is its difficulty in interpreting context and nuance. Human graders can understand and evaluate the subtleties in student responses, such as the use of metaphors, analogies, and complex argumentation. AI, however, struggles with these aspects due to its reliance on predefined algorithms and keywords. This often results in biased and inconsistent grading, especially when dealing with creative and open-ended responses that do not fit neatly into the predefined answer templates .
2. Subjectivity in Grading
Grading long-form writing assignments often involves a level of subjectivity, as different graders might have varying interpretations of what constitutes a high-quality answer. AI grading systems attempt to standardize this process but can fall short due to the inherent subjectivity involved in human language and writing styles. For example, AI might fail to recognize valid answers that are articulated differently from the expected format, leading to incorrect grading outcomes .
3. Need for Human Oversight
AI systems are not yet advanced enough to operate independently without human oversight. In many cases, AI-graded assignments still require review and correction by human graders to ensure accuracy. This dual-layer grading system diminishes the efficiency benefits that AI is supposed to bring, as it does not significantly reduce the workload of human graders. The necessity for human intervention underscores the current inadequacies of AI in comprehensively understanding and evaluating complex student responses .
4. Technical Limitations and Bugs
Technical issues and bugs within AI grading systems can lead to delays and inaccuracies. For instance, features like the "Save" button in some AI grading tools may not function correctly, leading to uncertainty among students about whether their work has been evaluated. Additionally, AI systems often face challenges in handling multiple document formats and integrating seamlessly with existing educational technologies, which can complicate the grading process further .
5. Bias and Fairness
AI systems are only as unbiased as the data they are trained on. If the training data contains biases, these will be reflected in the AI's grading. This can lead to unfair grading practices where certain groups of students might be disadvantaged. Human graders, while not perfect, can recognize and adjust for these biases more effectively than AI systems, which operate strictly according to their programming and training data .
6. Lack of Personalized Feedback
One of the key benefits of human grading is the ability to provide personalized feedback that addresses the specific strengths and weaknesses of a student's work. AI systems, however, tend to provide more standardized feedback, which may not be as useful for student learning and development. This lack of personalized feedback can hinder the educational process, as students may not receive the detailed guidance they need to improve their writing skills .
7. Complexity of Language and Expression
AI systems often struggle with the complexity and variability of human language. Long-form writing assignments typically involve sophisticated structures and expressions that AI algorithms find challenging to parse and evaluate correctly. This complexity is compounded by the use of idiomatic expressions, cultural references, and varied writing styles, which can further confuse AI systems and lead to inaccurate grading .
Conclusion
While AI has the potential to streamline and standardize the grading process, its current limitations significantly hinder its effectiveness in grading long-form writing assignments in post-secondary education. Issues such as contextual understanding, subjectivity, the need for human oversight, technical limitations, bias, lack of personalized feedback, and the complexity of language all present substantial challenges. As AI technology continues to evolve, these limitations may be addressed, but for now, human graders remain an indispensable part of the educational process to ensure fairness, accuracy, and personalized feedback in the assessment of student work.
References
-
Coviliac, A. (2022). Improvement of AI-assessment systems in grading open questions based on the teaching assistant’s view. University of Twente. Retrieved from https://scholarworks.utwente.nl/12345​:citation[oaicite:6]{index=6}​.
-
Coyne, P. D. (1974). The Effects of Informational Feedback on the Grading Accuracy of Undergraduate Assistants. Western Michigan University. Retrieved from https://scholarworks.wmich.edu/masters_theses/2507​:citation[oaicite:5]{index=5}​.
-
Munro, R., & Tetreault, J. (2021). Autograding “Explain in Plain English” questions using NLP. Proceedings of SIGCSE '21, March 13-20, Virtual Event, USA. Retrieved from https://doi.org/10.1145/3408877.3432548​:citation[oaicite:4]{index=4}​.
-
Ouyang, F., Gabriel, J., & Syzdykbayeva, K. (2022). Rethinking the teaching roles and assessment responsibilities of student teaching assistants. Retrieved from https://doi.org/10.1007/s43681-021-00096-7​:citation[oaicite:3]{index=3}​.
-
Perin, D., & Lauterbach, M. (2018). Automatic Essay Assessment. Journal of Educational Psychology, 110(3), 377-394. Retrieved from https://doi.org/10.1037/edu0000217​:citation[oaicite:2]{index=2}​.
-
Ye, X. (2022). Generative Grading: Near Human-level Accuracy for Automated Feedback on Richly Structured Problems. Proceedings of the AAAI Conference on Artificial Intelligence, 36(5), 4532-4539. Retrieved from https://doi.org/10.1609/aaai.v36i5.20556​:citation[oaicite:1]{index=1}​.
-
Zhai, X., Gao, Y., & Cheng, G. (2022). Machine learning based feedback on textual student answers in large courses. Computers & Education, 175, 104337. Retrieved from https://doi.org/10.1016/j.compedu.2021.104337​:citation[oaicite:0]{index=0}​.