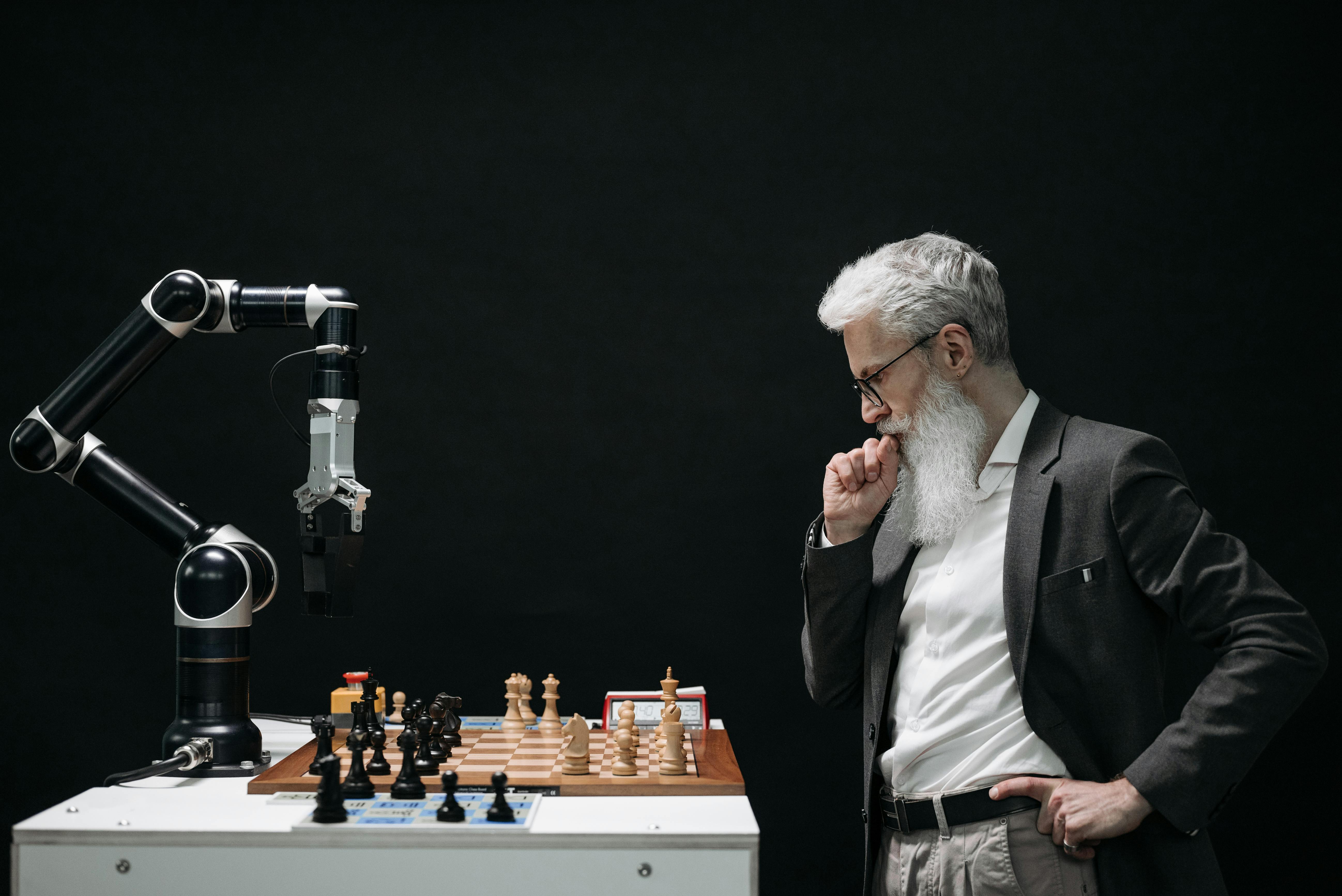
The newest trend in the world of artificial intelligence is Humanization, a term meant to indicate that a particular tool can produce outputs that more closely resemble what a real person would produce, and thus are harder to detect as being the work of AI.
As an educator, humanized AI outputs might seem like a new challenge to authenticating student submissions. After all, if AI tools suddenly begin producing work that's more human in tone, content, and accuracy - surely that will make it harder to distinguish?
Not quite - as with most things about AI, it's easy for companies to brand rudimentary AI functionality or moderate advances in the technology as a substantial leap forward. Is AI getting better at producing human-like work? Without question. Are "AI Humanizer" solutions a radical departure from what students are already using? No.
This is your chance to learn more about how AI tools and Large Language Models (LLMs) function, and optimize their outputs to better mimic human language. Read on to get better acquainted.
How to Define What Sounds Human
LLM's are trained on a wide variety of materials, and while there are genuine concerns about the possibility of AI submissions being used to train new AI tools, most of what current LLM's are trained on were originally written by humans. In theory this should lead to human sounding responses, right? Not exactly, because the translation from linguistic to mathematical and back again (the core of all human AI interactions) adds a layer of complexity that has to be accounted for. So while most LLM responses are human-like, there is often a stilted or slightly off element that gives it away as being AI generated, and that's what humanization claims to solve.
In order to deliver more natural sounding responses from their AI tools, developers have had to get reacquainted with elements of human language in its most technical form. These are six of the most common elements AI is being trained to incorporate into its responses:
-
Natural Language Proficiency
Fluency: Responses are grammatically correct and fluid, resembling human conversation.
Context Awareness: The ability to understand and appropriately respond to the context of the conversation. -
Empathy and Emotional Intelligence
Emotion Recognition: Identifying and responding to the emotional tone of the user.
Empathetic Responses: Providing responses that show understanding and empathy, making the interaction feel more personal and caring. -
Personalization
Adaptability: Tailoring responses to individual users based on previous interactions and known preferences.
Relevance: Providing responses that are directly relevant to the user's needs and interests. -
Conversational Coherence
Consistency: Maintaining a coherent thread throughout the conversation, avoiding contradictory statements.
Memory: Recalling past interactions to build continuity in ongoing dialogues. -
Humor and Creativity
Wit and Humor: Using appropriate humor to make interactions more enjoyable and engaging.
Creative Responses: Generating responses that are imaginative and can provide unique perspectives or solutions. -
Cultural and Social Awareness
Cultural Sensitivity: Understanding and respecting cultural differences and norms.
Social Cues: Recognizing and appropriately responding to social cues and etiquette.
Staying Ahead of the Curve
When an LLM incorporates these elements it can output responses that are much closer to human work, but we have yet to reach a point where the results are consistently flawless. This is especially apparent when students neglect to actually review an AI's work before turning it in as their own. Here are some things to look for in student submissions that might give away AI generated content:
- Consistency in Writing Style: Uniform tone and style throughout the assignment, lacking a distinct personal voice.
- Depth of Analysis: Surface-level analysis with predictable arguments, lacking critical thinking and nuanced insights.
- Specificity and Relevance: Generic examples and analogies that are not specific to course material or personal experiences.
- Coherence and Flow: Mechanical transitions and overly structured logical flow.
- Grammar and Syntax: Perfect grammar and syntax that may not match the student's usual writing style.
- Originality: Lack of original thought and potential plagiarism.
- Feedback Integration: Absence of specific feedback incorporation from previous assignments.
- Cultural and Emotional Sensitivity: Misplaced cultural references and inappropriate emotional tone.
Academic Misconduct, or New Way of Working?
A 2023 study recently showed that more than half of all college students have used AI on assignments or exams. That percentage is only going to increase as younger generations matriculate from more lax academic settings like high school with AI tools under their belt.
Some faculties and schools have started relying on AI tools to combat academic misconduct, with mixed results. Plagiarism, one of the clearest forms of cheating AI can check for, is a clear example of academic misconduct. But students may have only generated part of their assignment with AI, or merely used it as a study aid, in which case not only will AI tools struggle to decisively identify AI's involvement, but your integrity office may not know definitively whether to classify the work as cheating or not.
It's clear that AI isn't going to stop being a challenge for professors - so the question is, how will you respond?
At EXAMIND we believe that AI can benefit both students and faculty when used correctly. It can save time, lead to better learning outcomes, and reduce the consternation often associated with the relationship between AI and Academic Integrity. Visit our website or book a demo today to discover how you can be among the premiere collection of educators working together to create a climate where AI strengthens academia.